Development economists have been using randomized controlled trials (RCTs) for the best part of two decades, 1 The Nobel Prize to Abhijit Banerjee, Esther Duflo and Michael Kremer was announced as this essay was being revised. As it already has done, the Prize will raise the visibility of the debate about the pros and cons of conducting RCTs directed towards economic development. The extensive press discussion has revealed substantive concerns, especially about ethics. It also reveals widespread misperceptions, among both critics and defenders, about how RCTs actually work, particularly highlighting the widespread but false beliefs that randomization guarantees that the treatment and control groups are similar prior to treatment, and that an RCT can demonstrates causality. and economists working on welfare policies in the US have been doing so for much longer. The years of experience have made the discussions richer and more nuanced, and both proponents and critics have learned from one another, at least to an extent. In this essay, I do not attempt to reconstruct the full range of questions that I have written about elsewhere. 2 Angus Deaton, 2007, “Instruments, randomization, and learning about development,” Journal of Economic Literature, 48 (2), 424–55. Angus Deaton and Nancy Cartwright, 2018, “Understanding and misunderstanding randomized controlled trials,” Social Science and Medicine, https://doi.org/10.1016/j.socscimed.2017.12.005. Angus Deaton, 2010, “Understanding the mechanisms of economic development,” Journal of Economic Perspectives, 24(3): 3–16. Instead, I focus on a few of the issues where it seems to me that misunderstandings are common, and I have some words about the ethics of RCTs in poor countries, about which I have not previously written.
RCTs are affected by the same problems of inference and estimation that economists have faced using other methods and no RCT can ever legitimately claim to have established causality.
The RCT is a useful tool, but I think that it is a mistake to put method ahead of substance. I have written papers using RCTs. 3 Angus Deaton, 2013, “The financial crisis and the wellbeing of Americans,” Oxford Economic Papers, 64(1), 1-26. Angus Deaton and Arthur A. Stone, 2016, “Understanding context effects for a measure of life evaluation: how responses matter,” Oxford Economic Papers, doi: 10.1093/oep/gpw022 Like other methods of investigation, they are often useful, and, like other methods, they have dangers and drawbacks. Methodological prejudice can only tie our hands. Context is always important, and we must adapt our methods to the problem at hand. It is not true that an RCT, when feasible, will always do better than an observational study. This should not be controversial, but my reading of the rhetoric in the literature suggests that the following statements might still make some uncomfortable, particularly the second: (a) RCTs are affected by the same problems of inference and estimation that economists have faced using other methods, and (b) no RCT can ever legitimately claim to have established causality.
My theme is that RCTs have no special status, they have no exemption from the problems of inference that econometricians have always wrestled with, and there is nothing that they, and only they, can accomplish. Just as none of the strengths of RCTs are possessed by RCTs alone, none of their weaknesses are theirs alone, and I shall take pains to emphasize those facts. There is no gold standard. There are good studies and bad studies, and that is all.
1. Are RCTs the best way of learning, or of accumulating useful knowledge?
Sometimes. Sometimes not. It makes no sense to insist that any one method is best, provided only that it is feasible. It has always seemed to me to be a mistake for J-PAL to do only RCTs, and thus leave itself open to the charge that it is more (or as) interested in proselytizing for RCTs than it is in reducing poverty. Though as Tim Ogden notes, 4 Timothy Ogden, 2020, “RCTs, the hype cycle, and a high plateau,” Chapter 5, in Florent Bédécarrats, Isabelle Guérin and François Roubaud, editors, Randomized controlled trials in the field of development: a critical perspective, Oxford University Press, forthcoming. the members of J-PAL use a wide range of techniques in their own work, so perhaps J-PAL is just the RCT wing of a broader enterprise. Martin Ravallion is exactly right when he argues 5 Martin Ravallion, 2020, “Should the randomistas (continue) to rule?” Chapter 2 in Florent Bédécarrats, Isabelle Guérin and François Roubaud, op cit. that the best method is always the one that yields the most convincing and relevant answers in the context at hand. We all have our preferred methods that we think are underused. My own personal favorites are cross-tabulations and graphs that stay close to the data; the hard work lies in deciding what to put into them and how to process the data to learn something that we did not know before, or that changes minds. An appropriately constructed picture or cross-tabulation can undermine the credibility of a widely believed causal story, or enhance the credibility of a new one; such evidence is “causal” in a more useful way than most papers with the word causal in the title. The art is in knowing what to show. But I don’t insist that others should work this way too.
The imposition of a hierarchy of evidence is both dangerous and unscientific. Dangerous because it automatically discards evidence that may need to be considered, evidence that might be critical. Evidence from an RCT gets counted even if when the population it covers is very different from the population where it is to be used, if it has only a handful of observations, if many subjects dropped out or refused to accept their assignments, or if there is no blinding and knowing you are in the experiment can be expected to change the outcome. Discounting trials for these flaws makes sense, but doesn’t help if it excludes more informative non-randomized evidence. By the hierarchy, evidence without randomization is no evidence at all, or at least is not “rigorous” evidence. An observational study is discarded even if it is well-designed, has no clear source of bias, and uses a very large sample of relevant people.
Economics is an open subject in the sense that good studies that produce new, important, and convincing evidence are usually judged on their merits. But it is good to be careful that merit not be a cover for methodological prejudice.
Hierarchies are unscientific because the profession is collectively absolved from reconciling results across studies; the observational study is wrong simply because there was no randomization. Such mindless neglect of useful knowledge is thankfully rare in economics, but there are many examples in other fields, such as medicine or education. Yet economists frequently do give special weight to evidence from RCTs based on methodology alone; such studies are taken to be “credible” without reference to the details of the study or consideration of alternatives.
Economics is an open subject in the sense that good studies that produce new, important, and convincing evidence are usually judged on their merits. But it is good to be careful that merit not be a cover for methodological prejudice. When I hear arguments that RCTs have proved their worth by producing good studies, I want to be reassured that the use of randomization is not itself a measure of worth and that the argument is not circular.
2. RCTs are rigorous and scientific
This rhetoric is rarely if ever justified. The adjectives are used as code words for RCTs. Frequently so. The rhetoric appears to be successful, at least with funders. It is often coupled with an appeal to the importance of RCTs in medicine, but rarely coupled with a realistic reading of the successes and failings of RCTs in medicine. In the US, drugs require positive RCTs in order to be licensed, yet prescription opioids, such as OxyContin, have killed hundreds of thousands of Americans in the last twenty years. There are differences between how RCTs work in social science and in medicine, a topic on which more thinking could usefully be done. On one occasion, I discussed a series of development trials with a senior funding manager of a large foundation. He was happy to admit that the results were limited in applicability, and that some of the results were likely incorrect, but was unimpressed. RCTs, after all, he told me, are more rigorous than any other method and for him, that was enough. I think he had a notion that rigour meant that the results were generalizable, or could be scaled up. Or perhaps he held the common belief that all other methods are worse. Being wrong did not appear to conflict with being rigorous.
That RCTs will identify what works to eliminate global poverty is a commendable but unfounded aspiration.
“Finding out what works” is another common rhetorical slogan that, at least judged by its repetition, is effective among the public. Nothing works except in context, and finding out what works where and under what circumstances is a real scientific endeavour. What works depends also on for whom and for what purpose; finding out what works is also a matter of values. There is no experiment or series of experiments that can answer such questions unconditionally. That RCTs will identify what works to eliminate global poverty is a commendable but unfounded aspiration.
3. Experimentation: kick it and see
I am all for experimentation.6 But there is no logical connection between experimenting and randomization. Indeed, one might be wise, when directing one’s kick, to be rather precise about one’s aim; kicking at random is not advisable, and it might hurt. The serious point here is that, in many cases, randomization is unhelpful for experimentation, it can turn a good experiment into a useless one. Information that we should be using to improve our study is scrambled.
There are many kinds of experiments where randomization is not required, or would obscure the results.
The key laboratory experiments in economics did not use randomization. 6 Andrej Svorenčik, 2015, The experimental turn in economics: a history of experimental economics, https://dspace.library.uu.nl/bitstream/handle/1874/302983/Svorencik.pdf?sequence=1&isAllowed=y The Industrial Revolution is often described as having come about by endless tinkering, not by randomization, which would have got in the way of purposeful trial and error. Another example I have used in the past 7 Angus Deaton, 2012, “Searching for answers with randomized experiments,” Development Research Institute, NYU, video presentation https://www.youtube.com/watch?v=yiqbmiEalRU (March 22, 2102) is the arcade video game, Angry Birds. The birds need to be fired at an angle from a catapult, and can sometimes be redirected, speeded up, or detonated in flight, the object being to kill the egg-stealing pigs that are hiding in inaccessible places. Given the immense number of combinations, a systematic set of RCTs would take unimaginably long, although a dexterous child can figure out the solution in minutes. There are many kinds of experiments where randomization is not required, or would obscure the results. Randomization, after all, is random and searching for solutions at random is inefficient because it considers so many irrelevant possibilities.
4. Small versus large
Lant Pritchett has provided a typically eloquent, funny, and passionate argument that it is growth that matters for poverty reduction, not “rigorous” (or not) project by project evaluation, whether of money or chickens. 8 Lant Pritchett, 2020, “Getting random right: a guide for the perplexed practitioner,” Chapter 3, in Florent Bédécarrats, Isabelle Guérin and François Roubaud, op cit. In Poor Economics, Abhijit Banerjee and Esther Duflo argue the opposite, that it is only at the level of the “small” that we know what we are doing, so we must build knowledge trial by randomized trial.
The debate is (at least) as old as the World Bank. Here is a simplified history. The Bank started out with the small, doing projects, ports, roads, power plants, and the like. It became quickly obvious that evaluating projects using commercial criteria often did not improve people’s lives, particularly in economies where prices were distorted by tariffs, marketing boards, rationing, or exchange controls. An early response by two groups of very distinguished economists was to develop shadow prices to replace the market prices. Partha Dasgupta, Stephen Marglin and Amartya Sen produced one set of methods for the United Nations, 9 Partha Dasgupta, Stephen Marglin, and Amartya Sen, 1972, Guidelines for project evaluation, United Nations Industrial Development Organization. and Ian Little and James Mirrlees another for the OECD. 10 Ian Little and James Mirrlees, 1974, Project appraisal and planning for developing countries, Basic Books. The latter was turned into a manual by Lyn Squire and Herman van der Tak for use in the World Bank. 11 Lyn Squire and Herman van der Tak, 1975, Economic analysis of projects, Johns Hopkins University Press for World Bank. Yet the calculations were sometimes elaborate, beyond the capabilities or inclinations of Bank lending officials whose own incentives were to move money quickly. And the rules must have seemed incomprehensible to policymakers in the countries asked to implement them. As an example of the primitive state of project evaluation in much of the world, Lyn Squire later noted 12 Lyn Squire, “Project evaluation in theory and practice,” in Chapter 21 in Hollis Chenery and T. N. Srinivasan, Handbook of Development Economics, Volume 2, pages 1126-27. that even the most elementary tool of project evaluation, the discounting of future benefits, was rarely used in borrowing countries left to themselves. (This was not the case in India, where economists in the Planning Commission meticulously calculated shadow prices; at least some swallowed their personal skepticism.) If the economy was comprehensively distorted, there was surely little point in evaluating projects at market prices, and evaluation at shadow prices was not a realistic alternative.
The remedy was to switch from the small to the large, to fix the distortions first, and to get the macroeconomy right before doing project evaluation. Structural adjustment was the result.
RCTs need a theory of implementation, or of scale up, that explains just how the results are to be used in practice. That has to include attention to unintended consequences...which are usually not included in the end-points of the trial.
In support of this, empirical analyses, like Pritchett’s, showed that economic growth was the way to generate material poverty reduction. The great episodes of material poverty reduction in the world—particularly China and India—were driven by economic growth and by globalization. Aggregate growth came with growth in the small too, more jobs, more opportunities, more roads, more and better schools and clinics, but those were seen as springing up more or less spontaneously in an economy with good institutions and where rapid growth was ongoing. None of this explained how to stimulate economic growth. For this, cross-country regressions could help. These were widely criticized and are easily mocked, but yielded some useful knowledge, such as the importance of domestic investment—certainly a key in China, India, or Korea—of the provision of public goods, and that foreign aid, even at its best, was not likely to do much to stimulate growth by itself. They also systematized and disciplined the evidence, which was better than the country by country anecdotes (aka war stories) that had dominated much of the previous discussion. But we learned more about what slows growth than what speeds it up. All valuable, but hardly the keys to eliminating poverty through faster growth. No one, as far as I am aware, suggested that RCTs were the key to economic growth; it is hard to tell a story in which RCTs had any relevance for poverty reduction in China. 13 Yao Yang, 2019, “The open secret of development economics,” Project Syndicate, Oct 22.
The Bank was half right. The better macroeconomic management in many countries around the world, the better understanding of monetary policy and central banking, as well as of the costs of exchange rate undervaluation and commodity price taxation have all contributed to better growth and poverty reduction, especially given time to operate. 14 William Easterly, 2019, “In search of reforms for growth: new stylized facts on policy and growth outcomes,” NBER Working Paper 26318, September. Economists today, adherents of the credibility revolution and of testing for causality, tend to dismiss such evidence on the grounds that, in their view, it is neither rigorous nor credible. (Yet they have no similar difficulty with the causal claim that RCTs are effective in reducing global poverty.)
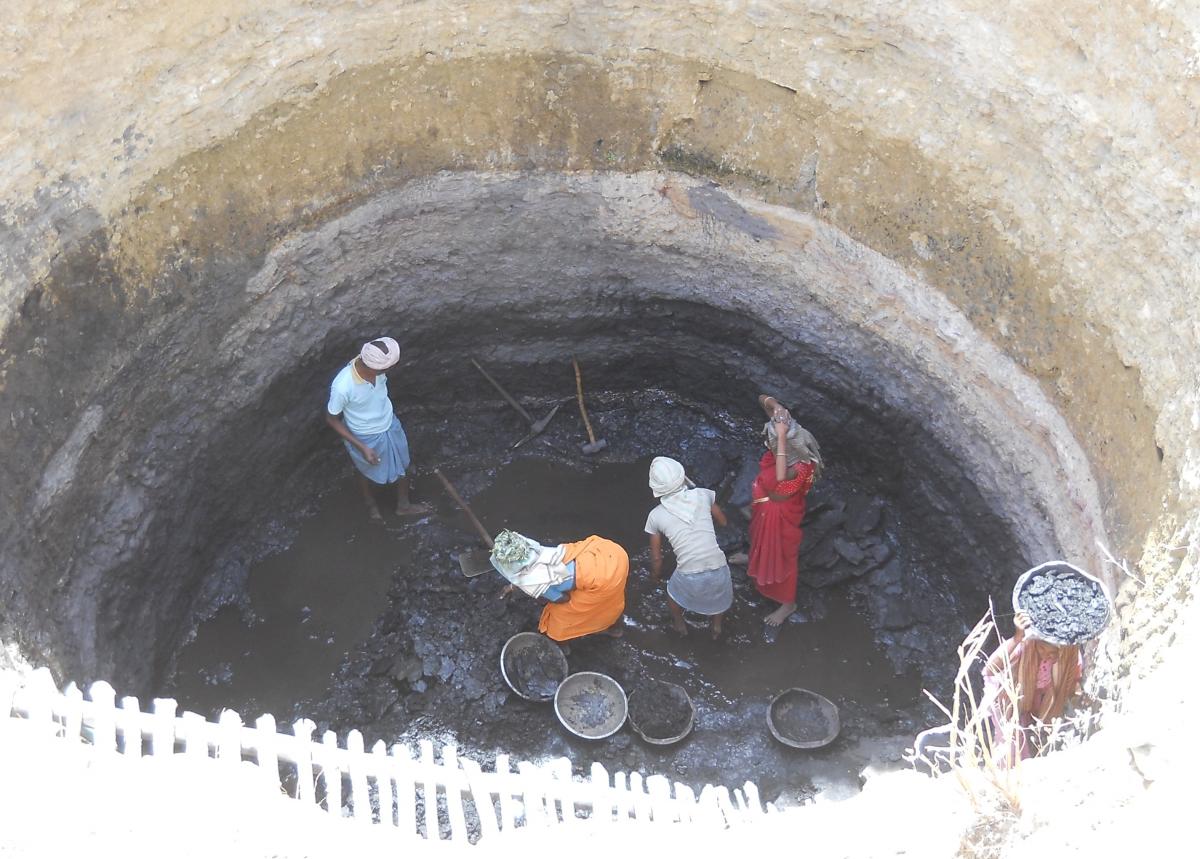
Those who believe that external help can aid economic development need to square the circle. No one doubts the importance of the macro perspective, only that the tools to influence economic growth are limited. The micro level trials are often successful in themselves, but their role in diminishing poverty rates is largely a matter of faith. RCTs need a theory of implementation, or of scale up, that explains just how the results are to be used in practice. That has to include attention to unintended consequences—the effects of implementation on the actions of government and communities—which are usually not included in the end-points of the trial. General equilibrium effects need to be thought through; scaling up will change prices and behaviors that were held constant in the experiments. RCTs routinely make the assumption that spill-over effects do not exist (the SUTVA assumption), yet the assumption is routinely violated, for example in sanitation 15 Dean Spears. Radu Ban and Oliver Cumming, 2020. “Trials and tribulations: the rise and fall of the sanitation RCT,” Chapter 7 in Florent Bédécarrats, Isabelle Guérin and François Roubaud, op cit. or deworming projects. At the individual level, the treatment works and spill-overs on others are small and often cannot be (or are not) measured. Yet, at the aggregate level, the sum of the individually small spill-overs can negate or reverse the effect.
5. Causality
A well-designed RCT will tell us something about causality. Yet, once again, there are many assumptions that need to be made to get from the data to the conclusion. In any finite trial, and there are no others, the possibility that the result is due to chance can never be ruled out. The measurement of the outcomes may matter, for example it is easy to design a case in which a treatment may improve income when income is measured in levels, but not when income is measured in logarithms. Yet most discussions seem to presuppose a concept of causality that is invariant to simple (monotone) transformations of the outcome that we are trying to affect. An RCT that establishes a positive average treatment effect implies only that at least one unit was positively affected; a majority of units may be affected negatively, or not at all. None of this seems to match the meaning of causality in common parlance, nor the enormous weight that economists today attach to what they call causal inference.
Alex Broadbent, Jan Vandenbroucke and Neil Pearce 16 Broadbent Alex, Jan P Vandenbroucke and Neil Pearce, 2017, “Formalism or pluralism? A reply to commentaries on `causality and causal inference in epidemiology,” International Journal of Epidemiology, 1-12. write “Causal conclusions do not follow deductively from data without a strong set of auxiliary assumptions, and these assumptions are themselves not deductive consequences of the data.” In the same paper they write, “we suggest that it is good practice to refrain from calling any individual study’s estimate `causal’ even if it is a randomized trial. It is the totality of the evidence that leads to the verdict of causality. Causality is a scientific conclusion, a theoretical claim, and as such transcends any individual study.” (italics added). Causality is in the mind, not in the data, an idea that Heckman and Pinto have traced back to Frisch and Haavelmo. 17 James J Heckman and Rodrigo Pinto, 2015. “Causal analysis after Haavelmo,” Econometric Theory, 31(1), 115-151. The triangulation of results, or learning about causal processes from many studies over time, is well-illustrated by the best work on sanitation in India. 18 Dean Spears. Radu Ban and Oliver Cumming, op cit.
An RCT that establishes a positive average treatment effect implies only that at least one unit was positively affected; a majority of units may be affected negatively, or not at all.
It is worth noting that it is not just the results of an RCT that may fail to transport, but causality itself. Nancy Cartwright and Jeremy Hardie 19 Nancy Cartwright and Jeremy Hardie, 2012, Evidence based policy: a practical guide to doing it better, Oxford. illustrate with a Rube Goldberg machine in which opening a window leads, through a long chain of preposterous but effective causal connections, to a pencil being sharpened by a woodpecker. Yet opening windows does not usually sharpen pencils, and a causal chain in one setting may be quite different in another setting. My impression is that when economists put the word “causal” in the titles of their paper, they are claiming more than a single instance in a specific context. Beware of Rube Goldberg.
That there are other ways of building causal models is well-known to economics students brought up in the Cowles tradition, or to readers of Judea Pearl. 20 Judea Pearl and Dana Mackenzie, 2018, The book of why: the new science of cause and effect, Basic Books. Pearl argues that we have to start with a causal model and then use it to confront the data and to test its structure and, like the Cowles Commission before him, offers a series of tools and methods to do so. The wisdom of Austin Bradford-Hill’s discussion 21 Bradford-Hill, Austin, 1965, “The environment and disease association or causation” Proceedings of the Royal Society of Medicine, 58, 295-300. of the many ways to detect causality seems to be little referred to in economics; Bradford-Hill was the pioneer in randomized clinical trials seventy years ago and it sometimes seems as if we are losing knowledge, not gaining it.
6. Ethics
It is important that economists should think about the ethics of experimentation. Yet some of the development RCTs seem to pose challenges to the most basic rules. How is informed consent handled when people do not even know they are part of an experiment? Beneficence is one of the basic requirements of experimentation on human subjects. But beneficence for whom? Foreign experimenters or even local government officials are often poor judges of what people want. Thinking you know what is good for other people is not evidence of beneficence.
Telling developing country policymakers that RCTs are the only way of gathering evidence for policy is unethical, because it can cause them to ignore important information.
Ethics also require us to be realistic about what RCTs can and cannot do. Ethical lapses are more easily justified for those who subscribe to the hierarchy view, that the only evidence that counts is evidence from RCTs, thus ruling out options that might pose fewer risks to subjects and might lead to better conclusions. Telling developing country policymakers that RCTs are the only way of gathering evidence for policy is unethical, because it can cause them to ignore important information.
My main concern is broader. Even in the US, nearly all RCTs on the welfare system are RCTs done by better-heeled, better-educated and paler people on lower income, less-educated and darker people. My reading of the literature is that a large majority of American experiments were not done in the interests of the poor people who were their subjects, but in the interests of rich people (or at least taxpayers) who had accepted, sometimes reluctantly, an obligation to prevent the worst of poverty, and wanted to minimize the cost of doing so. 22 Judith Gueron and Howard Rolston, 2013, Fighting for reliable evidence, Russell Sage. That is bad enough, but at least the domestic poor get to vote, and are part of the society in which taxpayers live and welfare operates, so that there is a feedback from them to their benefactors. Not so in economic development, where those being aided have no influence over the donors. Some of the RCTs done by western economists on extremely poor people in India, and that were vetted by American institutional review boards, appear unethical, sometimes even bordering on illegality, and likely could not have been done on American subjects. 23 Ankur Sarin, 2019, “Indecent proposals in economics,” The India Forum, Nov 1. https://www.theindiaforum.in/article/indecent-proposals-economics It is particularly worrying if the research addresses questions in economics that appear to have no potential benefit for the subjects. Using poor people to build a professional CV should not be accepted. Institutional review boards in the US have special protection for prisoners, whose autonomy is compromised; there appears to be no similar protection for some of the poorest people in the world. There is an uncomfortable parallel here with the debates about pharmaceutical countries testing drugs in Africa.
The technocrats believe that they can develop other people’s countries from the outside, because they know how to find out what works.
I see RCTs as part of what Bill Easterly calls the “technocratic illusion” 24 William Easterly, 2013, The Tyranny of Experts: economists, dictators, and the forgotten rights of the poor, Basic Books. that is the original sin of economic development, an aspect of what James Scott 25 James C. Scott, 1998, Seeing like a state: how certain schemes for improving the human condition have failed, Yale has called “high modernism,” that technical knowledge, even in the absence of full democratic participation, can solve social problems. According to this doctrine, which seems especially prevalent in Silicon Valley, among foundations, and in the effective altruism movement, global poverty will yield to the right technical fixes, one of which is the adoption of RCTs as the basis for evidence-based policy. Ignoring politics is seen as a virtue, not the vice that it is. Foundations and altruists often “know” what is good for poor people, and have the best intentions, but provide little evidence that poor people agree with their assessments or value their remedies, so that their interests can easily come to conflict with those they are trying to help. The technocrats believe that they can develop other people’s countries from the outside, because they know how to find out what works. In this, at least, there is no great difference between designing a gadget and designing social policy. Both are exercises for engineers.
Engineering poverty reduction is at best hopeless, and at worst disastrous. Development agencies today use the word “partnership” a great deal, but there is no genuine partnership when all the money is on one side. Nor can there be genuine informed consent in an RCT when aid money is at stake.
Social plumbing should be left to social plumbers, not experimental economists who have no special knowledge, and no legitimacy at all.
Finding out what works is not the same thing as finding out what is desirable. Good intentions by donors are no guarantee of desirability. Jean Drèze has provided an excellent discussion of the issues of going from evidence to policy 26 Jean Drèze, 2018, “Evidence, policy, and politics,” Ideas for India, August 3. https://www.ideasforindia.in/topics/miscellany/evidence-policy-and-politics.html .One of his examples is the provision of eggs to schoolchildren in India, a country where many children are inadequately nourished. An RCT could be used to establish that children provided with eggs come to school more often, learn more, and are better nourished. For many donors and RCT advocates, that would be enough to push for a “school eggs” policy. But policy depends on many other things; there is a powerful vegetarian lobby that will oppose it, there is a poultry industry that will lobby, and another group that will claim that their powdered eggs—or even their patented egg substitute—will do better still. Dealing with such questions is not the territory of the experimenters, but of politicians, and of the many others with expertise in policy administration. Social plumbing should be left to social plumbers, not experimental economists who have no special knowledge, and no legitimacy at all. 27 Esther Duflo, 2017, “The economist as plumber,” American Economic Review, 107(5), 1-26.
Working to benefit the citizens of other countries is fraught with difficulties. In countries ruled by regimes that do not care about the welfare of their citizens—extractive regimes that see their citizens as source of plunder—the regime, if it has complete control, will necessarily be the beneficiary of aid from abroad. This is most obvious in war zones where it is impossible to deliver aid without paying off the warmongers and prolonging or worsening the suffering. 28 Alex de Waal, 1997, Famine crimes: politics and te disaster relief industry in Africa, Currey. The dilemma extends to peacetime too. In authoritarian regimes with full control, it is only possible for outsiders to help when it is in the government’s interest to accept that help. Development agencies then find themselves in the situation of being “allowed” to help the poor, or to help provide health services, while providing political cover for the “enlightened” despot who is thereby free to persecute or eliminate his opponents. 29 Angus Deaton, 2015, “The logic of effective altruism,” Boston Review. http://bostonreview.net/forum/logic-effective-altruism/angus-deaton-response-effective-altruism Similar issues arise in democracies too, though less sharply; the step from evidence to policy is never ethically neutral but is less fraught when the poor have a voice and some political power.
What does this have to do with RCTs? Irrelevance for one. It makes no sense to spend resources randomizing schools or medicines when the President, facing an election, is imprisoning his foes or inciting violence against his tribal and political enemies. 30 Michela Wrong, 2009, It’s our turn to eat: the story of a Kenyan whistleblower, Harper. As larger numbers of the world’s poor come to live in nominally democratic states with populist autocratic leaders, more and more ethical dilemmas will confront trialists. Why are agencies funding aid, or RCTs to support aid, in countries whose leaders do not accept the liberal democratic beliefs of the donors, or experimenters? I am not claiming there are no answers to this question, only that donors need to know what they are.
There have already been protests 31 Sabah Hamid, 2019, “Why I resigned from the Gates Foundation,” New York Times, September 26. “Dismay at Gates Foundation prize for Narendra Modi,” The Guardian, Letter, 23 September, 2019, “Bill and Melinda Gates Foundation under fire for award to Narendra Modi,” The Guardian, 12 September 2019. about the Bill and Melinda Gates Foundation’s award of one of its Global Goal Awards to Narendra Modi for building toilets in India at a time when Modi is depriving Kashmiris of their rights, threatening to deprive millions of Assamese of their citizenship, and showing a preference for using religion as a criterion to confer citizenship on immigrants. The Foundation argues that the reward recognizes only Modi’s achievements in sanitation; this is surely a perfect example of limitations and dangers of technocratic aid. It empowers despotism and intolerance. Modi has received other prestigious awards from development agencies, including the United Nations. And much worse has happened repeatedly in Africa.
Aid agencies are turning a blind eye to political repression so long as the oppressors help check off one the Sustainable Development Goals, preferably as demonstrated by randomized controlled trials. The RCT is in itself a neutral statistical tool but as Dean Spears notes, 32 Dean Spears, personal communication, October 14, 2019. Quoted with permission. “RCTs provide a ready and high-status language” that allows “mutual legitimization among funders, researchers, and governments.” When the RCT methodology is used as a tool for “finding out what works,” in a way that does not include freedom in its definition of what works, then it risks supporting oppression.
(This is an extract and minimal revision from my paper in Florent Bédécarrats, Isabelle Guérin and François Roubaud, editors, Randomized controlled trials in the field of development: a critical perspective, Oxford University Press, forthcoming. For generous and helpful comments on an earlier version, I am most grateful to Nancy Cartwright, Anne Case, Shoumitro Chatterjee, Nicolas Côté, Jean Drèze, William Easterly, Reetika Khera, Lant Pritchett, Dean Spears and Bastian Steuwer. The views expressed here are my own.)